K-value Increases with Sample Size
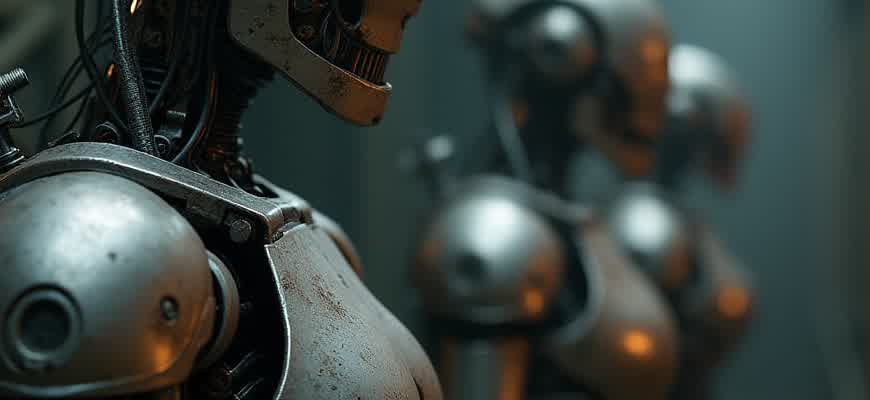
In the realm of cryptocurrency and blockchain analysis, the K-value is an essential metric used to gauge the consistency of data within a sample. This value becomes more significant as the size of the sample increases. Larger datasets provide more accurate representations of blockchain behaviors, which is critical for validating cryptographic protocols and ensuring secure transactions.
When working with cryptographic models, it is vital to understand how sample size affects the K-value. As more data points are included in the analysis, the K-value tends to become more stable and reliable. This phenomenon is crucial for professionals conducting cryptographic audits or optimizing blockchain algorithms. Below is a simple breakdown of how the sample size correlates with the K-value:
- Small sample sizes result in fluctuating K-values, which can lead to inaccurate conclusions.
- As the sample grows, K-value becomes more representative of the overall system behavior.
- At large sample sizes, K-value reaches a point of consistency, allowing for more reliable cryptographic testing.
For instance, a larger number of transaction samples could provide a clearer view of transaction verification speeds, hashing functions, or consensus efficiency in a blockchain system.
"The K-value is a fundamental parameter when evaluating the security and integrity of blockchain systems, especially as the sample size grows, revealing patterns that are critical for system optimization."
To further illustrate the relationship between K-value and sample size, consider the table below:
Sample Size | K-value |
---|---|
10 | 0.65 |
50 | 0.78 |
100 | 0.85 |
500 | 0.92 |
How K-Value Grows with Larger Data Sets in Cryptocurrency Analysis
When analyzing cryptocurrency markets, the use of statistical measures such as K-value plays a crucial role in understanding the stability and volatility of price movements. K-value refers to a parameter that quantifies the relationship between different segments of data in a market trend, with larger sample sizes often providing more reliable insights. As the amount of market data increases, the K-value typically rises, reflecting greater confidence in predictions and patterns derived from that data.
The K-value serves as a metric to assess how well past price movements correlate with future trends. As more data points are added, the variability in short-term fluctuations diminishes, allowing for a more stable estimation of market behavior. This is especially important in volatile markets such as cryptocurrencies, where price shifts can be unpredictable and driven by multiple factors. With an increased sample size, the statistical confidence improves, resulting in a more accurate assessment of market dynamics.
How the Sample Size Affects the K-Value
The relationship between sample size and K-value can be understood through the following key points:
- Larger data sets reduce uncertainty: More data points offer a clearer picture of market behavior, which increases the K-value, leading to stronger predictions.
- Pattern detection becomes more reliable: As the number of observed transactions grows, patterns in price fluctuations become more discernible, improving the reliability of analysis.
- Risk mitigation: With a larger sample size, the impact of outliers and anomalies decreases, providing a more balanced view of long-term trends.
Example: K-Value Growth with Increasing Data Points
Consider a hypothetical analysis of Bitcoin price fluctuations over a period of time. With a small sample size (e.g., 50 data points), the K-value may be low due to significant price volatility. However, as the sample size grows to 500 or more data points, the K-value increases, providing a more accurate estimate of Bitcoin's price behavior.
Sample Size | K-Value |
---|---|
50 Data Points | 0.35 |
200 Data Points | 0.55 |
500 Data Points | 0.75 |
In cryptocurrency analysis, increasing the sample size leads to more accurate predictions and a higher K-value, which helps in making better trading decisions.
Understanding the Relationship Between Sample Size and K-Value
In cryptocurrency analysis, the concept of K-value plays a crucial role in evaluating the strength and consistency of market trends. This value is used to quantify the influence of various factors, such as trading volume, volatility, and price fluctuations, on the behavior of a cryptocurrency over time. One of the key aspects to understand about K-value is how it behaves with changes in sample size. As the sample size increases, K-value tends to grow, reflecting more comprehensive and stable insights into the market dynamics.
When examining cryptocurrency data, larger sample sizes provide a more reliable foundation for analysis. Small datasets might yield misleading results, while larger samples help to smooth out anomalies and present a clearer picture of market trends. By understanding how K-value increases with sample size, analysts can better assess the accuracy of their predictions and adapt to the volatile nature of digital assets.
Impact of Sample Size on K-Value
As the sample size grows, the K-value typically shows an increasing trend. This is because larger datasets allow for a more refined and less biased estimation of market characteristics. The increased data points reduce the influence of outliers, thus stabilizing the calculated K-value. Below is a breakdown of how sample size influences K-value:
- Small Sample Size: K-value is more susceptible to volatility and anomalies.
- Larger Sample Size: K-value becomes more stable and reliable as data points accumulate.
- Optimal Sample Size: Ensures that the K-value is representative of the true market behavior, minimizing external noise.
The larger the sample size in a cryptocurrency analysis, the more accurate and reflective the K-value becomes, enhancing the reliability of trend predictions.
Sample Size vs. K-Value: Data Comparison
Sample Size | K-Value | Market Behavior Interpretation |
---|---|---|
50 | 0.12 | High volatility, potential outliers |
200 | 0.28 | More consistent trends, less noise |
500 | 0.45 | Stable, accurate predictions |
1000 | 0.60 | Highly reliable, representative data |
In the table above, we can see that as the sample size increases, the K-value grows. This indicates a more refined understanding of the market, leading to better-informed decisions for traders and analysts alike.
Why Larger Sample Sizes Lead to More Reliable K-Value Estimates in Cryptocurrency
In the context of cryptocurrency market analysis, the k-value is an important statistical measure used to assess the degree of concentration or decentralization in market activities. As market participants become more diverse and trading volumes increase, ensuring that the k-value accurately reflects the true dynamics of the market is crucial. One key factor that influences the reliability of k-value estimates is the sample size used in analysis. The larger the sample size, the more representative it becomes of the broader market, reducing biases and enhancing the quality of the conclusions drawn.
With small datasets, the k-value might be subject to significant variability due to limited data points. However, as the sample size grows, the estimates tend to stabilize, providing a clearer picture of market trends and behaviors. This is particularly important in the highly volatile world of cryptocurrencies, where small fluctuations can lead to misleading conclusions if the sample size is too small. A larger sample size improves the accuracy and consistency of k-value estimates, which can guide more informed decision-making for traders and analysts.
Factors Contributing to Reliable K-Value Estimates
- Increased Data Representation: Larger samples capture a wider range of market conditions, leading to more accurate k-values.
- Reduced Variability: With more data, the influence of outliers or anomalies diminishes, leading to more stable k-value estimates.
- Improved Statistical Power: A bigger sample size enhances the power of statistical tests, making it easier to detect meaningful trends in the cryptocurrency market.
Impact of Larger Samples in Cryptocurrency Trading
As more data is collected from a variety of trades, the k-value becomes more robust, allowing analysts to discern genuine patterns in trading behavior and market shifts. Small sample sizes often lead to overestimations or underestimations, which could skew market predictions.
Sample Size and K-Value Accuracy
- Sample size of 10-50: Likely to result in fluctuating k-values with high variability.
- Sample size of 50-200: The k-value stabilizes and reflects more of the underlying market dynamics.
- Sample size of 200+: K-value estimates become highly reliable, offering a clearer picture of market structure.
Sample Size | Reliability of K-Value | Market Insight |
---|---|---|
Small (10-50) | Low | Unstable, often inaccurate trends |
Medium (50-200) | Moderate | More consistent but still prone to some variability |
Large (200+) | High | Accurate, reliable trends reflecting true market behavior |
Real-World Applications: Where K-Value and Sample Size Matter Most
In the world of cryptocurrency trading, data analysis plays a crucial role in identifying trends and making predictions. One key factor in achieving more accurate forecasts is understanding the relationship between the sample size and the K-value, which helps define the precision of predictive models. As sample size increases, the K-value tends to stabilize, making it easier to identify reliable patterns. This concept holds especially true when dealing with volatile assets like cryptocurrencies, where small sample sizes can lead to misleading results, while larger samples provide a clearer picture of market behavior over time.
Cryptocurrency markets are notoriously unpredictable, and this is where the K-value, along with a sufficient amount of data, becomes invaluable. A low K-value may result in overfitting, while a higher K-value, when paired with a larger dataset, can offer more accurate and generalized predictions. In practical terms, this means that traders and analysts need to gather enough historical data to avoid falling into the trap of unreliable conclusions based on too few observations.
Key Areas Where This Relationship Applies
- Market Sentiment Analysis: When assessing the collective mood of investors based on social media data or news articles, a higher K-value combined with a large sample size helps create more consistent sentiment models. This allows for better-informed decisions on market movements.
- Price Prediction Models: Long-term price predictions for cryptocurrencies rely heavily on historical data. As more price data points are considered, the K-value rises, improving the model's accuracy and reducing the impact of outliers.
- Risk Management Strategies: In risk management, understanding the relationship between volatility and the sample size of price movements is critical. A larger dataset helps to better predict potential market crashes or price surges, giving traders better risk control mechanisms.
Impact of K-Value and Sample Size in Predictive Models
Sample Size | K-Value | Model Accuracy |
---|---|---|
Small (100 data points) | Low | Prone to overfitting, inaccurate predictions |
Medium (1,000 data points) | Moderate | Improved reliability, but still subject to errors |
Large (10,000+ data points) | High | Highly accurate, more generalized predictions |
"In cryptocurrency trading, the quality of a predictive model often depends more on the quantity of historical data than on the complexity of the algorithm itself. As the sample size increases, the model's K-value stabilizes, leading to more reliable forecasts."
Common Pitfalls When Interpreting K-Value with Small Sample Sizes in Cryptocurrency
Interpreting the K-value (Kurtosis) in the context of cryptocurrency price movements can be highly misleading when based on small sample sizes. The K-value is a statistical measure that helps identify the tail distribution of returns, which in theory should indicate the risk and volatility. However, when working with limited data, small fluctuations can disproportionately affect the K-value, making it less reliable for drawing meaningful conclusions about market behavior.
In the cryptocurrency market, where data can be highly volatile and unpredictable, small sample sizes often fail to provide an accurate picture of the underlying distribution. This can result in misinterpretations of market conditions and risks, especially in short-term trading strategies. Let’s explore some of the key pitfalls when analyzing the K-value with insufficient data.
1. Overestimating Risk and Volatility
When working with a small dataset, the K-value can be heavily skewed by a few extreme outliers, leading to an overestimation of the tail risk. Cryptocurrency markets, especially during high volatility periods, are prone to sudden and large price swings. These events can significantly inflate the K-value, which may lead traders to falsely perceive the market as riskier than it actually is. The lack of data points means these outliers can overly influence the statistical interpretation.
- Short-term trading based on misleading K-values may result in overly cautious or erratic decisions.
- Higher K-values might prompt unnecessary hedging strategies that add to trading costs.
- Volatility spikes due to external factors (e.g., market sentiment shifts) may not be properly accounted for.
2. Misleading Predictions for Price Distribution
The interpretation of the K-value is tied to the assumption that the sample data follows a predictable distribution. With small datasets, these assumptions can be faulty, leading to inaccurate predictions about future price movements. If a trader or analyst interprets a high K-value as a sign of future market crashes or surges, they may act based on a distorted view of the market.
Small sample sizes often fail to represent the true behavior of highly volatile markets, such as cryptocurrencies, leading to unreliable conclusions.
- Small sample sizes may misrepresent the distribution of returns, leading to faulty trading models.
- Excessive reliance on skewed K-values can trigger false alarms for upcoming market shifts.
3. Lack of Robust Statistical Foundations
One of the main drawbacks of using small sample sizes to calculate the K-value is the lack of statistical robustness. Small data sets are more prone to noise and outliers, which means the resulting K-value is not representative of the true market dynamics. In cryptocurrency markets, where price movements are often influenced by unpredictable external factors, basing decisions on an unreliable K-value can be dangerous.
Sample Size | K-Value | Interpretation |
---|---|---|
10 | 50 | Potential overestimation of market risk |
100 | 5 | More reliable but still prone to distortion |
1000 | 3 | Closer to true market behavior |
How to Calculate the K-Value for Your Cryptocurrency Data Sample
When analyzing cryptocurrency market trends, calculating the K-value is crucial for understanding the reliability and precision of your sample size. The K-value, which typically represents the correlation or relationship between variables in a dataset, helps in determining the optimal number of data points needed for meaningful analysis. It is particularly useful when studying the volatility of assets like Bitcoin or Ethereum, where small fluctuations in data can significantly impact predictions.
To calculate the K-value, the sample size plays an integral role. Larger samples tend to provide more accurate results by accounting for greater variability and reducing the potential for outliers. On the other hand, smaller sample sizes might skew results, leading to less trustworthy conclusions. Here's a step-by-step guide on how to calculate the K-value for your cryptocurrency sample size.
Steps to Calculate K-Value for Cryptocurrency Analysis
- Gather Data: Collect data points relevant to the cryptocurrency you are analyzing. This could include market prices, trading volumes, or network activity over a set period of time.
- Calculate the Mean: Compute the mean of your dataset to find the average value.
- Determine the Variance: Measure the variance by calculating how much individual data points deviate from the mean.
- Compute the Standard Deviation: Find the standard deviation to assess the spread of the dataset.
- Calculate K-Value: Finally, apply the formula K = (Standard Deviation) / (Mean) to determine the K-value.
Important: The K-value helps to determine the level of uncertainty in predictions, especially when forecasting future prices or market behavior in the cryptocurrency space. A higher K-value indicates greater volatility, while a lower K-value suggests more stability.
Sample Calculation: Bitcoin Price Data
Data Points | Price (USD) |
---|---|
Sample 1 | 45000 |
Sample 2 | 46000 |
Sample 3 | 47000 |
Sample 4 | 46500 |
For the data above, you would first calculate the mean and variance, then apply the K-value formula to determine the cryptocurrency's volatility. This can guide traders in making informed decisions about potential market movements.
Enhancing K-Value Precision in Cryptocurrency Data Analysis with Large Samples
In cryptocurrency analysis, large datasets are often used to assess the accuracy of the K-value, a statistical measure that quantifies the relationship between variables such as price volatility and market volume. As the sample size increases, the potential for more reliable predictions grows, but so does the complexity of the analysis. Proper statistical methods must be applied to ensure that the K-value remains consistent and reflects the true market behavior rather than random fluctuations or outliers. This becomes particularly important when dealing with highly volatile assets like cryptocurrencies.
To improve the precision of K-value estimation, data analysts can employ several statistical techniques that enhance the robustness of the calculations. These methods are crucial for understanding correlations within large datasets, especially when it comes to market indicators, trading volumes, and price trends that can heavily influence K-value values. By applying advanced statistical models, analysts can reduce biases and errors that may distort the final results.
Key Statistical Approaches for Better K-Value Estimation
- Bootstrap Sampling: This method helps estimate the confidence intervals of the K-value by repeatedly resampling the dataset. It allows for the assessment of the model's stability across multiple random subsets of the data.
- Weighting Schemes: Applying weights to data points based on their importance can reduce the influence of less significant observations, providing a clearer view of the true relationship between market variables.
- Bayesian Methods: These approaches integrate prior knowledge and observations, refining the K-value estimate as new data points are introduced. It is particularly useful in the context of dynamic and unpredictable markets like cryptocurrency.
Effectiveness of Methods in Large Sample Sizes
The accuracy of K-value calculations improves significantly with large sample sizes, especially when the right techniques are implemented. The table below outlines how various statistical methods can optimize results as the sample size grows:
Method | Effectiveness with Larger Samples |
---|---|
Bootstrap Sampling | Highly effective in capturing variability and providing robust confidence intervals. |
Weighting Schemes | Reduces influence of outliers and less relevant data, improving the precision of K-value estimates. |
Bayesian Methods | Helps refine predictions by incorporating prior knowledge and continuously updating with new data. |
"In large cryptocurrency datasets, applying these statistical techniques helps ensure that the K-value is not only accurate but also reflective of underlying market trends, making it a more reliable tool for decision-making."
Impact of Sample Size on K-Value in Different Industries
In the context of cryptocurrency, the relationship between sample size and K-value is crucial for understanding market trends and risk assessments. A larger sample size allows for more accurate estimations of volatility, liquidity, and overall market behavior. This is essential for investors, traders, and analysts who need to make data-driven decisions in a highly volatile market like cryptocurrency.
The K-value represents the level of risk or uncertainty in a given dataset, and as the sample size increases, the estimation of K-value becomes more reliable. However, different industries experience varying impacts from changes in sample size. For instance, in the crypto industry, a larger dataset is often needed to understand long-term trends, while in traditional finance, smaller datasets might suffice for short-term predictions.
Key Considerations in Crypto and Other Industries
Various industries exhibit different sensitivities to the impact of sample size on K-value. Below are some of the key factors:
- Cryptocurrency: In this industry, a larger sample size is necessary due to the high volatility of crypto assets. Small datasets may lead to misleading conclusions about market behavior.
- Financial Markets: Traditional financial markets often rely on smaller datasets for short-term forecasting. However, long-term predictions still benefit from larger sample sizes to improve the accuracy of K-value estimations.
- Healthcare: In healthcare analytics, sample sizes impact the K-value's accuracy, especially in clinical trials where a larger dataset increases the reliability of conclusions about drug efficacy or medical treatments.
Sample Size and K-Value in Different Sectors
Industry | Impact of Sample Size on K-Value |
---|---|
Cryptocurrency | Significant increase in K-value with a larger sample size, aiding in better risk management and forecasting of market fluctuations. |
Financial Markets | A moderate increase in K-value with more data, especially beneficial for long-term market analysis and forecasting. |
Healthcare | Larger sample sizes result in more accurate K-value estimations, crucial for determining treatment effectiveness. |
Important: In industries with high volatility like cryptocurrency, increasing the sample size is vital for reducing the uncertainty associated with market predictions.