Quant Crypto Use Case
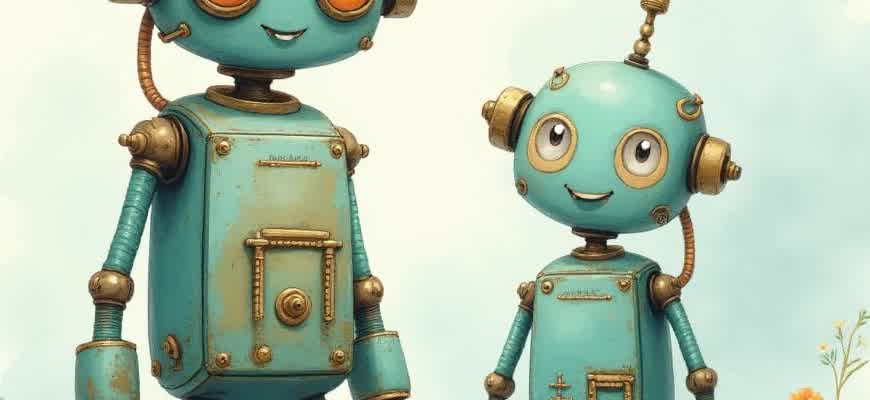
Quant Network offers a unique solution to the blockchain interoperability challenge. Its innovative technology connects different blockchains, facilitating seamless communication and data transfer between disparate networks. One of the main advantages of Quant is its Overledger OS, which allows developers to create multi-chain applications without the need for deep blockchain expertise. Below are key features of Quant's functionality in the crypto space:
- Cross-chain Interoperability: Enables smooth interaction between various blockchain protocols, providing a unified network.
- Scalability: Quant's architecture supports high transaction throughput, helping to reduce congestion across networks.
- Security: The platform maintains robust security standards for both developers and end-users through its advanced cryptographic methods.
In the realm of decentralized finance (DeFi), Quant also plays a pivotal role by enabling the transfer of assets across different platforms, significantly enhancing liquidity and reducing friction in trading. This is especially beneficial for institutions looking to tap into DeFi ecosystems without the technical complexities associated with blockchain development. The table below highlights some of the primary use cases:
Use Case | Description |
---|---|
Tokenization | Allows assets to be tokenized and moved between different blockchain platforms. |
Cross-chain Smart Contracts | Facilitates the creation of smart contracts that operate across multiple blockchains. |
DeFi Interoperability | Bridges liquidity and assets between different DeFi platforms, optimizing trade efficiency. |
"Quant Network's Overledger is a game changer for blockchain integration, enabling frictionless communication across multiple chains without compromising security."
Optimizing Trading Strategies with Quantitative Models in Crypto
In the rapidly evolving world of cryptocurrency, effective trading strategies are paramount for success. Traditional methods often fall short when applied to the high volatility and fast-paced nature of digital asset markets. Quantitative models, however, provide a structured approach to trading by leveraging mathematical and statistical techniques to analyze and predict price movements. These models can be used to optimize trading decisions, reduce risks, and improve profitability in cryptocurrency markets.
One of the key advantages of quantitative crypto models is their ability to process large amounts of data quickly. By identifying patterns and trends from historical data, these models offer traders a more reliable basis for decision-making. This is especially valuable in the cryptocurrency market, where even small market shifts can lead to significant gains or losses. Implementing these models allows traders to execute automated strategies that can outperform manual trading, particularly in terms of speed and accuracy.
Key Aspects of Quantitative Crypto Trading Models
- Data-Driven Decisions: Quantitative models rely on vast amounts of historical and real-time data to identify trading signals.
- Risk Management: These models are equipped to analyze risk and develop strategies to minimize potential losses through position sizing and stop-loss algorithms.
- Automation: Trading strategies are often automated, allowing for faster execution of orders and minimizing human error.
- Backtesting: Before deployment, strategies can be backtested against historical data to assess their viability in different market conditions.
Quantitative crypto models offer a strategic advantage by continuously analyzing market conditions and executing trades based on preset criteria, allowing traders to optimize their performance without constant manual intervention.
Examples of Common Quantitative Models
- Mean Reversion: This model assumes that asset prices tend to revert to their historical mean over time. It identifies overbought or oversold conditions and trades accordingly.
- Momentum Trading: Based on the principle that assets with upward momentum will continue to rise, and those with downward momentum will continue to fall.
- Machine Learning Models: These models use algorithms to detect complex patterns in large datasets, often outperforming traditional statistical methods.
Performance Evaluation
Model | Risk Factor | Profit Potential | Backtest Success Rate |
---|---|---|---|
Mean Reversion | Medium | Moderate | 75% |
Momentum Trading | High | High | 80% |
Machine Learning | Low | High | 85% |
Integrating Quantitative Techniques into Cryptocurrency Portfolio Strategy
As cryptocurrency markets continue to mature, integrating quantitative analysis into portfolio management has become a crucial step for optimizing returns and mitigating risks. Quantitative methods help investors make data-driven decisions by utilizing mathematical models, statistical analysis, and automated strategies. These techniques allow portfolio managers to assess market conditions, track performance, and predict future trends with higher precision, reducing the emotional bias often associated with trading decisions.
Portfolio managers increasingly rely on quantitative strategies such as algorithmic trading, risk assessment models, and predictive analytics to enhance their cryptocurrency holdings. By incorporating historical data, price volatility, and market sentiment, these methods provide a more robust approach to navigating the complexities of the crypto market. The integration of quantitative techniques empowers investors to develop more structured and scalable investment strategies, ultimately aiming for better risk-adjusted returns.
Key Quantitative Approaches in Crypto Portfolio Management
- Algorithmic Trading: Automated trading systems that execute predefined strategies based on quantitative signals such as moving averages or momentum indicators.
- Risk Parity: Balancing portfolio risk by allocating assets based on their volatility and correlation with other assets.
- Mean Reversion: Analyzing price deviations and betting on a return to historical averages, often used in volatile markets like cryptocurrencies.
Steps to Implement Quantitative Analysis in Crypto Portfolios
- Data Collection: Gather historical price data, on-chain metrics, and market sentiment to identify key trends.
- Model Development: Build mathematical models using techniques like regression analysis or machine learning to predict price movements and optimal allocations.
- Backtesting: Test the models on historical data to evaluate their effectiveness and fine-tune the strategies for real-world application.
- Risk Management: Incorporate stop-loss, position sizing, and portfolio rebalancing rules to manage risks dynamically.
"Quantitative analysis in crypto markets offers a systematic approach that reduces the emotional volatility of trading, enabling data-driven decision-making."
Advantages of Quantitative Analysis in Crypto Portfolios
Benefit | Explanation |
---|---|
Improved Risk Management | Quantitative models can dynamically adjust the portfolio to minimize exposure to high-risk assets, protecting investors during market downturns. |
Increased Market Efficiency | Algorithmic strategies can quickly capitalize on short-term price discrepancies, often before they are recognized by human traders. |
Backtested Performance | By testing models with historical data, portfolio managers can gain insights into potential future performance and optimize strategies accordingly. |
Reducing Risk Exposure through Algorithmic Crypto Trading
In the rapidly fluctuating world of cryptocurrency, risk management is crucial for traders seeking to minimize potential losses. Algorithmic trading, using advanced computational models, provides a way to manage these risks effectively. By leveraging automated strategies, traders can ensure that their decisions are based on data-driven insights rather than emotional reactions, which are common in volatile markets.
These algorithms are designed to analyze market data, identify trends, and execute trades in real-time, allowing for quicker and more precise decision-making. The main advantage is their ability to mitigate human errors, enhance consistency, and adhere to predefined risk parameters. This approach provides an edge in navigating high-risk environments like crypto trading, where volatility can be both a challenge and an opportunity.
Key Benefits of Algorithmic Trading in Crypto
- Automated Risk Control: Algorithms are programmed to monitor market conditions and act when specific thresholds are met, ensuring exposure is limited.
- Speed and Precision: Automated systems can execute trades far faster than humans, ensuring that opportunities are seized instantly, reducing the risk of slippage.
- Consistency: Trading bots do not suffer from emotional biases or fatigue, making their strategies more reliable over time.
"Algorithmic trading enables traders to implement risk management rules that are difficult to execute manually, such as setting stop-loss or take-profit orders with precision."
Example of Risk Reduction Through Algorithmic Strategy
Strategy | Risk Reduction Method |
---|---|
Trend Following | Minimizes losses by only entering trades in the direction of the prevailing market trend. |
Mean Reversion | Limits risk by taking positions when the market deviates too far from its average, assuming a return to the mean. |
Arbitrage | Reduces exposure by exploiting price differences between markets, ensuring low-risk, high-reward trades. |
Conclusion
By using algorithmic trading, crypto traders can significantly reduce their risk exposure while capitalizing on market inefficiencies. These systems offer an efficient and reliable way to implement complex strategies, ensuring that decisions are consistent and based on objective market data rather than subjective judgment.
How Quantitative Models Drive Price Prediction in Crypto Markets
In the volatile world of cryptocurrencies, predicting price movements can be particularly challenging due to market unpredictability and external factors. However, quantitative models offer a systematic approach to analyzing large datasets and making forecasts based on historical trends, technical indicators, and statistical patterns. These models utilize advanced algorithms to process and analyze information from various sources, helping traders and investors identify trends that may not be immediately obvious through traditional analysis.
Quantitative models are designed to minimize human bias by relying on objective data and well-established mathematical techniques. They offer insights into price dynamics by leveraging methods such as time series analysis, machine learning, and algorithmic trading strategies. These models allow for a deeper understanding of market behaviors and help to identify optimal entry and exit points for trading, reducing emotional decision-making and improving trading efficiency.
Key Quantitative Techniques for Price Prediction
- Time Series Analysis: This method involves analyzing historical price data to predict future price trends. By looking at patterns in past data, time series models can forecast potential future price movements.
- Machine Learning Algorithms: Machine learning models can be trained on vast amounts of historical data to predict future outcomes. These models continuously improve as they process more data, making them adaptable to changes in the market.
- Statistical Arbitrage: Statistical arbitrage strategies focus on price inefficiencies between different crypto assets. By using mathematical models, traders can capitalize on price discrepancies that exist momentarily in the market.
Practical Applications of Quantitative Models
- Automated Trading: Quantitative models are often used in algorithmic trading systems, which execute buy and sell orders automatically based on predefined criteria derived from the models' predictions.
- Risk Management: By accurately predicting price movements, quantitative models help traders and investors manage risk by determining the optimal amount to invest in each trade and setting stop-loss orders to minimize losses.
- Market Sentiment Analysis: Quantitative models can also incorporate social media data and news sentiment analysis to gauge market sentiment and adjust predictions accordingly.
Impact of Quantitative Models on Crypto Markets
"Quantitative models provide a systematic framework for analyzing the unpredictable nature of crypto markets, allowing traders to make data-driven decisions rather than relying on speculation."
By using quantitative methods, crypto traders can gain a competitive edge in navigating market complexities. As the market continues to mature, these models are expected to become even more advanced, integrating more diverse data sources and refining their prediction capabilities. Through these tools, cryptocurrency trading may become increasingly automated and efficient, offering a clearer path to profitability for both individual traders and institutional investors.
Model | Use Case |
---|---|
Time Series Forecasting | Prediction of future price movements based on historical data |
Machine Learning | Identifying complex patterns in price data for more accurate predictions |
Statistical Arbitrage | Exploiting price inefficiencies in crypto markets |
Utilizing Machine Learning for Crypto Arbitrage and Market Making
The rapid evolution of cryptocurrency markets has given rise to new opportunities and challenges for traders. Machine learning (ML) techniques have become essential tools for both arbitrage strategies and market making. By leveraging the vast amounts of data generated in crypto markets, algorithms can make decisions faster and more accurately than human traders, leading to improved profitability and reduced risks. In the context of arbitrage, ML models can identify price inefficiencies across different exchanges, allowing for swift execution of trades that capitalize on price discrepancies. Similarly, for market making, ML can predict price trends and liquidity gaps, ensuring optimal buy and sell orders are placed with minimal slippage.
Machine learning enhances both the speed and accuracy of executing these strategies by analyzing vast datasets, considering historical patterns, and responding to real-time market movements. These capabilities are particularly valuable in volatile markets like cryptocurrencies, where traditional manual trading strategies often struggle to keep up. The following outlines some of the ways ML is transforming crypto arbitrage and market making.
Machine Learning in Crypto Arbitrage
- Price Prediction Algorithms: Machine learning models can forecast price movements based on historical data, trading volume, and market sentiment, enabling arbitrage traders to anticipate price discrepancies between exchanges.
- Real-time Data Analysis: ML algorithms continuously analyze multiple exchange feeds, identifying arbitrage opportunities within milliseconds, which is crucial in the highly volatile crypto space.
- Automated Trade Execution: Once an arbitrage opportunity is detected, the system can automatically execute trades, ensuring faster reactions than manual interventions.
Machine Learning in Market Making
- Order Book Prediction: ML algorithms can analyze order book data to predict future price movements and potential liquidity gaps, optimizing market making strategies.
- Risk Management: Machine learning helps to dynamically adjust bid-ask spreads based on market volatility, reducing risk while maintaining profitability.
- Sentiment Analysis: Sentiment analysis tools powered by machine learning assess market sentiment from news, social media, and trading data, providing insights into future price movements.
Machine learning's ability to process and analyze vast amounts of data in real time makes it indispensable for arbitrage and market making in the fast-moving cryptocurrency markets.
Example: Market Making Using Machine Learning
Feature | Machine Learning Benefit |
---|---|
Real-time Order Book Analysis | Predicts liquidity imbalances, adjusts strategies on the fly. |
Automated Risk Management | Minimizes exposure during high volatility by adjusting spread. |
Sentiment Analysis | Incorporates social media signals to refine market predictions. |
Applying Quantitative Techniques to Manage Crypto Market Volatility
The volatile nature of cryptocurrency markets presents a unique set of challenges for investors and traders. Quantitative strategies, powered by advanced mathematical models and algorithms, offer a sophisticated approach to navigating this uncertainty. By utilizing historical data, real-time analytics, and predictive modeling, quantitative techniques allow market participants to optimize risk management and enhance decision-making processes, helping them hedge against sharp price movements and unexpected market shifts.
One of the most effective uses of quantitative methods in crypto trading involves portfolio optimization and volatility forecasting. These strategies not only help in identifying high-risk periods but also in determining the most appropriate hedging assets. By continuously analyzing vast amounts of data, they provide actionable insights that can significantly reduce exposure to adverse market conditions.
Key Strategies for Hedging Crypto Volatility
- Volatility Forecasting: This technique focuses on predicting future price swings based on historical patterns and current market data. By using models like GARCH (Generalized Autoregressive Conditional Heteroskedasticity), traders can estimate the level of market volatility and adjust their positions accordingly.
- Correlation Analysis: Understanding the relationships between different assets can help in diversifying portfolios. Quantitative models use correlation metrics to find assets that move in opposite directions to crypto, thereby reducing overall portfolio risk.
- Options and Derivatives: Crypto options and futures contracts are used to hedge against potential price declines. By purchasing put options or entering short futures positions, investors can protect themselves from sudden price drops.
Tools for Implementing Quantitative Hedging Strategies
Strategy | Tool/Technique | Benefit |
---|---|---|
Volatility Forecasting | GARCH models, Monte Carlo simulations | Predicts market volatility and adjusts positions to reduce risk |
Correlation Analysis | Statistical correlation metrics, machine learning algorithms | Identifies safe assets for diversification, balancing the portfolio |
Options & Derivatives | Crypto options, futures contracts | Provides protection against extreme price moves |
"In volatile markets, quantitative techniques provide an edge by turning data into actionable insights, making it possible to adjust risk exposure proactively."
Enhancing Market Liquidity and Trade Efficiency through Algorithmic Strategies in Cryptocurrencies
The cryptocurrency market, known for its high volatility and fragmented liquidity, presents challenges in executing large trades without significant slippage. Quantitative trading algorithms have proven to be highly effective in overcoming these obstacles, providing traders with more efficient ways to execute transactions while minimizing market impact. These algorithms leverage complex mathematical models to predict market trends, optimize order placement, and enhance liquidity by facilitating smoother price discovery.
By implementing algorithmic strategies, market participants can improve the execution quality of their trades, achieve better pricing, and manage risk more effectively. This is especially crucial in markets like crypto, where liquidity can be sparse in certain assets, and the speed of execution directly influences profitability. Below are key approaches that quantify liquidity and execution efficiency in the crypto market:
Key Algorithmic Approaches to Improving Liquidity and Execution
- Market Making: Algorithms continuously place buy and sell orders, contributing to liquidity and narrowing bid-ask spreads.
- Smart Order Routing: These systems analyze multiple exchanges and automatically direct orders to the venue with the best price and liquidity.
- Slippage Control: Algorithms adjust trade sizes and timing to minimize slippage, ensuring that the price at which an order is executed remains close to the expected price.
"Quantitative algorithms optimize liquidity provision, helping to stabilize markets and reduce transaction costs for traders."
Algorithm Performance Metrics
Metric | Description |
---|---|
Execution Speed | Time taken to execute an order from initiation to completion. |
Price Impact | The degree to which an order affects the market price. |
Slippage | The difference between the expected execution price and the final execution price. |
Liquidity Depth | The amount of liquidity available at different price levels in the order book. |